我们先从消息队列说起。消息队里本质上就是一个pub-sub 发布订阅的一个模式。这里有三个角色
技术其实基本都是来源于生活的场景的。我记得大学期间去玩具厂打暑假工的时候。我被分配到其中一个组里面。
一个玩具的制作不是全部由一个人完成的,而是每一个人做一部分,最后组合成为一个完整的玩具:当时是所有人排成一个队列,然后前面就是一个传送带。上游的人做完之后,就会放到传送带里面传送给下一个人继续组装。
上游和下游速度不匹配:这里会有一个问题,由于每一部分的难度和工人的娴熟度不一样,往往会造成互相等待的情况。比如我是暑假工,不是很娴熟,干活的速度自然就会慢点,而我上游是一个老员工,手脚麻利做的很快。每次我前面都堆了一大堆半成品,不得不催上面的大哥:搞不完了,大哥慢点。这个时候大哥就停下来跟我唠嗑。你看,这样我的速度就会影响到大哥的速度,继而影响整个产品的进度。
通过半成品暂存,来减少等待现象:工厂的计算钱的方式是看你完成的数量的。一开始大哥还是可以体谅的,在我干不完的时候停下来等我,和我唠嗑,偶尔也帮我搞下,但是人家大哥也是来赚钱的,不是来和我唠嗑的。后面大哥就建议我把半成品先搬到地下暂存着,我慢慢搞,他继续做,这样大哥就不用停下来等我了,等到后面大哥想休息了过来帮我或者我自己加班把这些慢慢消化掉。
上面这个例子对应的消息队列的概念:
所以我们可以得出,消息队列解决几个问题:
秒杀是一个永远绕不开的一个技能表演的场景,你技术行不行就看你能不能扛得住一个高并发的秒杀就知道了。就拿秒杀业务来讲,tps 峰值在1w/s。一个秒杀业务涉及的库存扣减,价格计算,订单落库。如果请求直接打到后端服务是扛不住的,因此我们需要一个消息队列,来暂存用户的请求消息,后端服务根据自己的消费速度从消息队列里面获取消息来进行消费。这种场景,消息队列就主要起到削峰填谷的作用,同时对消息队列提出一个新的要求:
从上面的一些使用场景和业务系统对消息队列能力的要求,我们不得不思考一个问题:我们的消息应该存储在哪里?
消息我们可以直接在内存中使用数组或者队列来存储数据即可。性能非常高。但是有几方面的缺点
既然要存储数据,就需要解决数据存哪里?从存储方式来看,主要有几个方面:
解决了存储之后,还要看需求是否满足,比如性能,吞吐量,本质上就是数据结构的设计决定的。我们看看上面数据存储方式对应的数据结构
# B-Tree vs LSM-Tree
B+tree
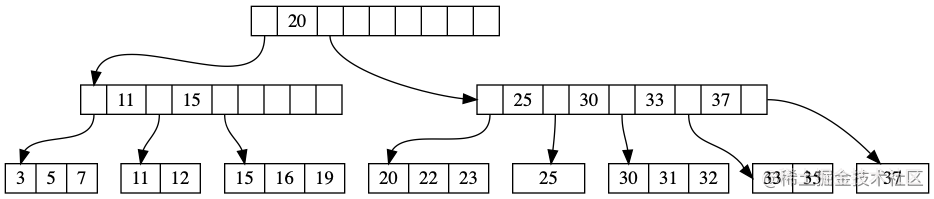
LSM
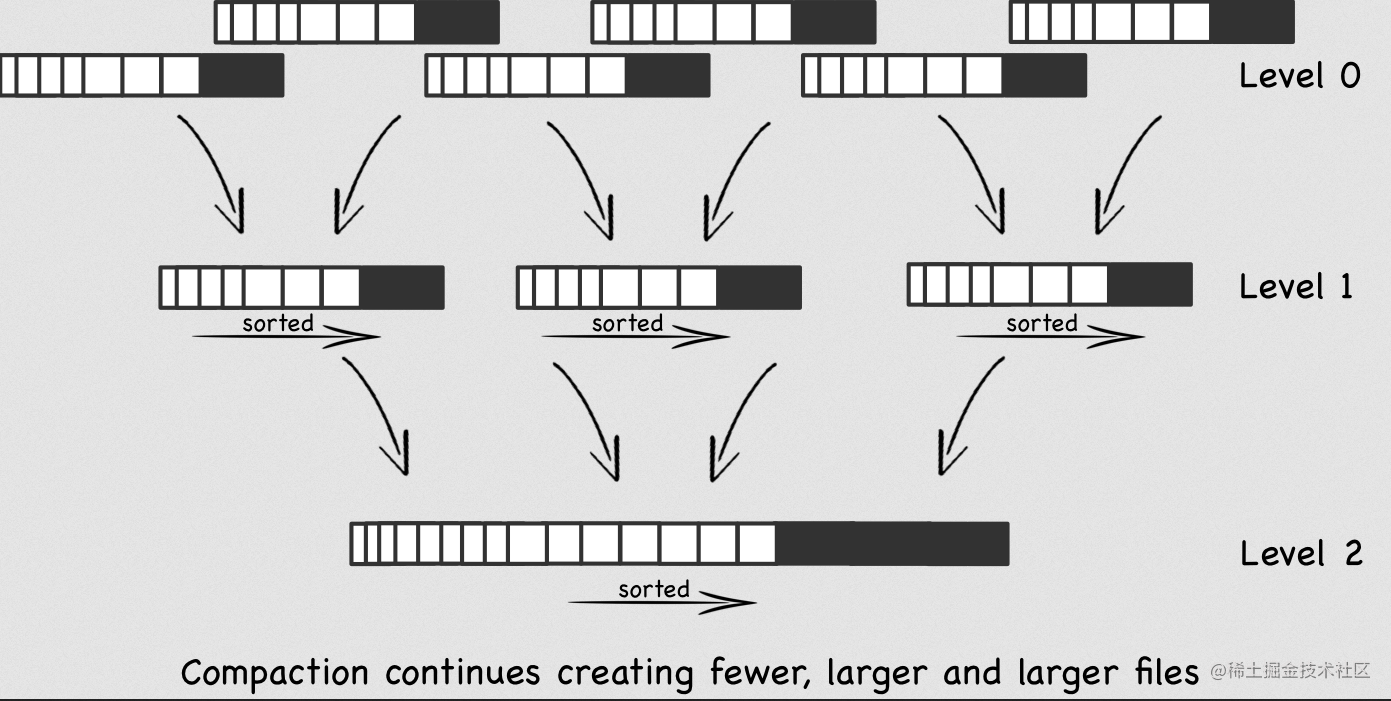
存储 | 数据结构 | 写性能 | 读 |
---|---|---|---|
mysql | B+ tree | 写一条数据需要两次写入 1、数据写入是按页为单位进行写的,假设页的大小为B 字节,那么写放大为Θ(B)(最坏的结果) 2、为了避免在写页的过程中出现故障,需要写入redo log(WAL) | 既支持随机读取又支持范围查找的系统。读放大为O(logBN/B),数据量大的适合性能会急剧下降,常规是b+ tree 超过4层,大约2000万记录是临界点 |
rocketdb | LSM tree | Memtable/SSTable实现,写的话也变成顺序写了(这一点是极大的优化点),但是后台会出现多路归并算法来合并,这个过程占用磁盘IO 会到当前消息的读写有扰动,写放大Θ(klogkN/B) | 读的顺序是MemTable->分层的sst ,性能会比B+ tree 略差,读放大Θ((log2N/B)/logk) |
文件系统 | append only log | 直接在文件末尾追加,所有的的写都是顺序的,因此性能极高 | 不支持根据内容进行检索,只能根据文件偏移量执行查询 |
mysql 在大数据量的情况,性能会急剧下降,并且扩展性非常不友好,从这一点是直接排除了mysql 了。
分布式KV 存储 天然的分布式系统,对大数据量和未来的扩展都问题不大,LSM tree 对写性能和吞吐都比mysql 要好。查的时候比mysql 差一点,查询其实是可以通过缓存等手段去做优化的,可以说是一个值得考虑选择。
但是,满足以上两点性能和吞吐量最优的毫无疑问是使用文件系统,因为消息不需要修改,读和写都是顺序读写,性能极高。
但是。。。但是现实中的需求更复杂一点,我们可能需要使用多个队列来完成不同的业务。比如一个队列来处理订单相关的业务,一个队列来处理商品相关的业务等等。那么我们该如何调整呢?我们都知道文件 append only log 的方式是不支持根据消息的内容来搜索的,所以如果所有的队列的数据存在一个文件中,显然没办法满足需求。换个思路,一个队列一个文件我们就可以绕开根据内容检索的需求。貌似也是没有问题,的确kafka 就是这么玩的。
思考一个问题,每个队列一个文件,那么读写还是顺序的吗?因为这个直接影响到性能 文件数量少的情况,大体还是顺序的
文件数量大,大体上就不是那么有序了
作为一款面向业务的高性能消息中间件,随着业务的复杂度变高,队列数量是急剧变大的。如果要保证写入的吞吐量和性能,还需要得所有的队列都写在同一个文件。但是,按照队列消费的场景就意味着要根据消息内容(队列名字)来进行消费,append only log 是不支持检索的,如何解决这个问题。想想我们在写sql 的时候慢了,我们为了提速就会增加一个索引。万事万物都是想通的,这里我们也可以根据建立一个队列的索引,每一个队列就是一个索引文件。读取数据的时候,先从索引队列找到消息在文件的偏移量后,在到数据文件去读取。这里你可能意识到了,索引文件的数量变大的之后,那么对索引文件的读写不就是又变成随机读写了吗?性能又会急剧下降?
um um 好问题。一个一个来解决:
方案 | 优点 | 缺点 |
---|---|---|
每一个queue 都单独一个文件 | 消费的时候不需要独立建立一个索引,系统复杂度降低,并且性能高 | 当queue 很多的时候,并且每个queue 的数据量都不是很大情况,就会存在很多小文件,写和读都讲变成随机读,性能会受到影响 |
所有queue 共享一个文件 | 所有的写都是顺序写的,性能比较高,可以支撑大量queue 性能也不至于下降的厉害 | 1、需要建立独立的索引文件,查询数据的链路变长,需要先从索引查到数据再到数据文件查询 2、索引队列本身也是小文件,好在因为数据量少,基本可以常驻内存 3、读变成随时读,不过整体还是顺序读 |
rocketmq 中数据文件称为:commitlog,topic索引文件称为 consumeQueue
结论:选择文件系统,append only log.根据消息队列即时消费和顺序读写的特点,刚写入的内容还在page cache ,就被读走了,甚至都不需要回到磁盘,性能会非常高。看看顺序读写的性能
如果所有的数据都存在一个commitlog 文件的话,如果数据量大了,这个文件必然会非常大,这样对性能就会有所影响,解决也很简单,我们大文件切换成小文件,每个文件固定大小1G,写满了就切换到一个新的文件
从上图可以看到,其实commitlog 是一个目录,下面挂着一些列大小相同的文件。每个文件都有一个名字,这里的名字就是取消息最小的offset 作为文件名字,由于每个文件大小都是一样的,也就意味着知道文件名字后 加上文件大小就可以知道这个文件存放消息的下标范围了。注意这里一个很重要的点消息的offset 是全局递增的,不再是具体文件的偏移量。这里就带出一个问题,我们从consumeQueue 取出来的是offset ,如何定位到具体的文件和当前文件的偏移量。假如上图的文件大小是2,那么我们要取offset =5的数据
- int index = (int) ((offset / this.mappedFileSize) - (firstMappedFile.getFileFromOffset() / this.mappedFileSize));
- 复制代码
-
- /**
- * Finds a mapped file by offset.
- *
- * @param offset Offset.
- * @param returnFirstOnNotFound If the mapped file is not found, then return the first one.
- * @return Mapped file or null (when not found and returnFirstOnNotFound is
false
). - */
- public MappedFile findMappedFileByOffset(final long offset, final boolean returnFirstOnNotFound) {
- try {
- MappedFile firstMappedFile = this.getFirstMappedFile();
- MappedFile lastMappedFile = this.getLastMappedFile();
- if (firstMappedFile != null && lastMappedFile != null) {
- if (offset < firstMappedFile.getFileFromOffset() || offset >= lastMappedFile.getFileFromOffset() + this.mappedFileSize) {
- LOG_ERROR.warn("Offset not matched. Request offset: {}, firstOffset: {}, lastOffset: {}, mappedFileSize: {}, mappedFiles count: {}",
- offset,
- firstMappedFile.getFileFromOffset(),
- lastMappedFile.getFileFromOffset() + this.mappedFileSize,
- this.mappedFileSize,
- this.mappedFiles.size());
- } else {
- // index 代表第几个文件,这里减去第一个文件的索引,因为文件是可能被回收的,所以要减去
- int index = (int) ((offset / this.mappedFileSize) - (firstMappedFile.getFileFromOffset() / this.mappedFileSize));
- MappedFile targetFile = null;
- try {
- targetFile = this.mappedFiles.get(index);
- } catch (Exception ignored) {
- // mappedFiles 是CopyOnWriteArrayList ,所以有可能存在并发的情况导致indexOuterOfException
- }
-
- if (targetFile != null && offset >= targetFile.getFileFromOffset()
- && offset < targetFile.getFileFromOffset() + this.mappedFileSize) {
- return targetFile;
- }
- // mappedFiles 是CopyOnWriteArrayList ,所以有可能存在并发的情况导致indexOuterOfException
- // 在上面异常的情况找不到,就需要遍历去查找了
- for (MappedFile tmpMappedFile : this.mappedFiles) {
- if (offset >= tmpMappedFile.getFileFromOffset()
- && offset < tmpMappedFile.getFileFromOffset() + this.mappedFileSize) {
- return tmpMappedFile;
- }
- }
- }
-
- // 这里依然有可能找不到,比如我们原本是要找第一个文件的,后面进来后刚好第一个文件因为过期被删除了,所以这里有可能被返回第一个文件
- if (returnFirstOnNotFound) {
- return firstMappedFile;
- }
- }
- } catch (Exception e) {
- log.error("findMappedFileByOffset Exception", e);
- }
-
- return null;
- }
- 复制代码
上文提到,消息队列的第一个特点就是数据量大,既然数据量大,数据如果只存在一个机器上的话,必然面临着瓶颈,因此我们需要把数据均衡的分发到各个机器上。思路其实也很简单,一段很长的队列平均切成N份,把这N份分别放到不同的机器上
从上图我们可以看到,上半部分的队列半平均切割成为2份,分别存储在机器A 和机器B上。同时可以看到左下角多了一个topicA 的来把机器A和机器B的两个队列关联起来,从原来的实际队列变成了一个抽象的逻辑队列(topic)
虽然我们的消息已经分成切分成为多份放到不同的机器了,但是每一份都是都只有一个副本,也就意味着,任何一台机器的硬盘坏掉的话,该机器上的消息就会丢失掉了,这对于钱大妈这种业务的系统是不可接受的。行业通常的做法一份数据存多个副本,并且确保所有的副本不能全都在同一台机器。问题来了,那么这多份数据是同步双写还是异步双写呢?
方案 | 优点 | 缺点 |
---|---|---|
同步双写 | 数据不会丢失 | 性能会降低,单个RT变长 |
异步双写 | 单个RT 更加小,性能更高,吞吐量更大 | 数据可能会丢失 |
其实每个业务场景需求是不一样的,RocketMq 是支持可配置的
之前我们一直聊的都是宏观上的设计方面,现在我们把视野聚焦到一些细节实现上.
我们先看下commitlog 的消息内容
这里重点解释下MAGICCODE 这个字段,还是有点意思的。因为commitlog 下面每个文件的长度是固定的,有没有想过这样一种场景,一条新的消息来了之后,但是刚好这个文件所剩余的空间不足以容纳下这条消息的时候,你会怎么做?一部分放在这个文件,另外一部分放在新的文件?如果是这样新的文件的名字是这条消息的offset 还是下条消息的offset?解析这条消息的时候就需要跨多个文件去读取,变得非常复杂,RocketMq 其实用的是另外一种方式,直接在最后面写入一个结束符号告诉用户结束了,后面的不用读取了,这种方式就比较简单了,虽然浪费一点点空间,但我觉得是值得的,业务消息本来并不大,并且已经限制了每条消息的大小了。这个标识结束的标识位就是放在MAGICCODE 来实现的,现在可以取两个值
- 普通消息 MESSAGE_MAGIC_CODE = -626843481
- 复制代码
- 文件结束符号 BLANK_MAGIC_CODE = -875286124
- 复制代码
- // Determines whether there is sufficient free space
- if ((msgLen + END_FILE_MIN_BLANK_LENGTH) > maxBlank) {
- // 进来就说明文件空间不足了,写上结束语就滚蛋了,返回去写下一个文件
- // 这里的设计不是直接写下一个文件,而是返回去重新进来写,保持逻辑统一,这个设计也是挺清晰的
- this.msgStoreItemMemory.clear();
- // 1 TOTALSIZE
- this.msgStoreItemMemory.putInt(maxBlank);
- // 2 MAGICCODE
- this.msgStoreItemMemory.putInt(CommitLog.BLANK_MAGIC_CODE);
- // 3 The remaining space may be any value
- // Here the length of the specially set maxBlank
- // 写完结束语可能还有剩,那也不管了,这样可能有点浪费空间,那也没办法啦,毕竟都是顺序写的,不可能再下一个小消息来的时候再回头写
- final long beginTimeMills = CommitLog.this.defaultMessageStore.now();
- byteBuffer.put(this.msgStoreItemMemory.array(), 0, 8);
- return new AppendMessageResult(AppendMessageStatus.END_OF_FILE, wroteOffset,
- maxBlank, /* only wrote 8 bytes, but declare wrote maxBlank for compute write position */
- msgIdSupplier, msgInner.getStoreTimestamp(),
- queueOffset, CommitLog.this.defaultMessageStore.now() - beginTimeMills);
- }
- 复制代码
org.apache.rocketmq.store.CommitLog.MessageExtEncoder#encode(org.apache.rocketmq.store.MessageExtBrokerInner)
- protected PutMessageResult encode(MessageExtBrokerInner msgInner) {
- this.byteBuf.clear();
- /**
- * Serialize message
- */
- final byte[] propertiesData =
- msgInner.getPropertiesString() == null ? null : msgInner.getPropertiesString().getBytes(MessageDecoder.CHARSET_UTF8);
-
- final int propertiesLength = propertiesData == null ? 0 : propertiesData.length;
-
- if (propertiesLength > Short.MAX_VALUE) {
- log.warn("putMessage message properties length too long. length={}", propertiesData.length);
- return new PutMessageResult(PutMessageStatus.PROPERTIES_SIZE_EXCEEDED, null);
- }
-
- final byte[] topicData = msgInner.getTopic().getBytes(MessageDecoder.CHARSET_UTF8);
- final int topicLength = topicData.length;
-
- final int bodyLength = msgInner.getBody() == null ? 0 : msgInner.getBody().length;
-
- final int msgLen = calMsgLength(msgInner.getSysFlag(), bodyLength, topicLength, propertiesLength);
-
- // Exceeds the maximum message
- if (bodyLength > this.maxMessageBodySize) {
- CommitLog.log.warn("message body size exceeded, msg total size: " + msgLen + ", msg body size: " + bodyLength
- + ", maxMessageSize: " + this.maxMessageBodySize);
- return new PutMessageResult(PutMessageStatus.MESSAGE_ILLEGAL, null);
- }
-
- // 1 TOTALSIZE
- this.byteBuf.writeInt(msgLen);
- // 2 MAGICCODE
- this.byteBuf.writeInt(CommitLog.MESSAGE_MAGIC_CODE);
- // 3 BODYCRC
- this.byteBuf.writeInt(msgInner.getBodyCRC());
- // 4 QUEUEID
- this.byteBuf.writeInt(msgInner.getQueueId());
- // 5 FLAG
- this.byteBuf.writeInt(msgInner.getFlag());
- // 6 QUEUEOFFSET, need update later
- this.byteBuf.writeLong(0);
- // 7 PHYSICALOFFSET, need update later
- this.byteBuf.writeLong(0);
- // 8 SYSFLAG
- this.byteBuf.writeInt(msgInner.getSysFlag());
- // 9 BORNTIMESTAMP
- this.byteBuf.writeLong(msgInner.getBornTimestamp());
-
- // 10 BORNHOST
- ByteBuffer bornHostBytes = msgInner.getBornHostBytes();
- this.byteBuf.writeBytes(bornHostBytes.array());
-
- // 11 STORETIMESTAMP
- this.byteBuf.writeLong(msgInner.getStoreTimestamp());
-
- // 12 STOREHOSTADDRESS
- ByteBuffer storeHostBytes = msgInner.getStoreHostBytes();
- this.byteBuf.writeBytes(storeHostBytes.array());
-
- // 13 RECONSUMETIMES
- this.byteBuf.writeInt(msgInner.getReconsumeTimes());
- // 14 Prepared Transaction Offset
- this.byteBuf.writeLong(msgInner.getPreparedTransactionOffset());
- // 15 BODY
- this.byteBuf.writeInt(bodyLength);
- if (bodyLength > 0)
- this.byteBuf.writeBytes(msgInner.getBody());
- // 16 TOPIC
- this.byteBuf.writeByte((byte) topicLength);
- this.byteBuf.writeBytes(topicData);
- // 17 PROPERTIES
- this.byteBuf.writeShort((short) propertiesLength);
- if (propertiesLength > 0)
- this.byteBuf.writeBytes(propertiesData);
-
- return null;
- }
- 复制代码
有了commitlog 了,我们还需要构建一个consumeQueue 来作为索引才能够供消费者使用,这个构建的过程是异步的,也就是数据保存到commitlog 成功后就可以直接返回给客户端了,服务器后台会启动一个线程一直在拉取commitlog 的消息出来进行构建consumeQueue.
从上图我们可以看出consumeQueue 每一条记录的长度是固定的,固定为20.为啥要固定呢? 消费者来consumeQueue 取消息的时候,拿到是consumeQueue的小标,而不是真实的offset,这个时候如果是定长才可能通过数据小标快速定位到消息的位置进行获取消息,如果是变长是没有办法实现的
tagsCode 的设计
消息的tag 是可选的,并且每个消息的tag长度还不一定相等,如果要维持consumeQueue 的记录是固定为20 的话,那就意味着不能直接存放tag 的内容,这里巧妙的设置为tag 的hashCode 。因为hash 是可能出现冲突的,意味着在服务端进行过滤消息的话可能会出现误判,把不该由消费者消费的消息发送给了消费者,因此消费者需要在本地做二次等值过滤,毕竟hash 冲突的可能性没有那么高,因此牺牲一点网络上的带宽换来读取消息的性能是值得的,从这里可以看出,架构上总是有取舍的,不是完美的。
- String tags = propertiesMap.get(MessageConst.PROPERTY_TAGS);
- if (tags != null && tags.length() > 0) {
- tagsCode = MessageExtBrokerInner.tagsString2tagsCode(MessageExt.parseTopicFilterType(sysFlag), tags);
- }
-
-
- public static long tagsString2tagsCode(final TopicFilterType filter, final String tags) {
- if (null == tags || tags.length() == 0) { return 0; }
-
- return tags.hashCode();
- }
-
- 复制代码
另外还有一个很有意思的是延迟消息,tagsCode 存放的是消息的延迟时间,这样就可以不用回到commitlog 就可以知道这个消息该什么时候投放给消费者,大大提高了性能
- // Timing message processing
- {
- /**
- * 延迟消息,消息队里的tagsCode 存在的是延迟的时间,这样在拿消息出来的时候可以快速的判断这个消息是否已经到了
- */
- String t = propertiesMap.get(MessageConst.PROPERTY_DELAY_TIME_LEVEL);
- if (TopicValidator.RMQ_SYS_SCHEDULE_TOPIC.equals(topic) && t != null) {
- int delayLevel = Integer.parseInt(t);
-
- if (delayLevel > this.defaultMessageStore.getScheduleMessageService().getMaxDelayLevel()) {
- delayLevel = this.defaultMessageStore.getScheduleMessageService().getMaxDelayLevel();
- }
-
- if (delayLevel > 0) {
- //这里对延迟消息做了个特殊处理,把延迟时间放到tagCode,牛逼,支持消费的时候快速过滤
- tagsCode = this.defaultMessageStore.getScheduleMessageService().computeDeliverTimestamp(delayLevel,
- storeTimestamp);
- }
- }
- }
-
-
- public long computeDeliverTimestamp(final int delayLevel, final long storeTimestamp) {
- Long time = this.delayLevelTable.get(delayLevel);
- if (time != null) {
- return time + storeTimestamp;
- }
-
- return storeTimestamp + 1000;
- }
-
- 复制代码
来看下consumeQueue 的构建源码,通过一个后台线程从commitlog 获取消息进行构建
- private void doReput() {
- if (this.reputFromOffset < DefaultMessageStore.this.commitLog.getMinOffset()) {
- log.warn("The reputFromOffset={} is smaller than minPyOffset={}, this usually indicate that the dispatch behind too much and the commitlog has expired.",
- this.reputFromOffset, DefaultMessageStore.this.commitLog.getMinOffset());
- this.reputFromOffset = DefaultMessageStore.this.commitLog.getMinOffset();
- }
- // 这里用一个doNext 变量来控制两个for 循环的结束(里面还嵌套一个for 循环),这个倒是可以借鉴下
- for (boolean doNext = true; this.isCommitLogAvailable() && doNext; ) {
-
- if (DefaultMessageStore.this.getMessageStoreConfig().isDuplicationEnable()
- && this.reputFromOffset >= DefaultMessageStore.this.getConfirmOffset()) {
- break;
- }
-
- // 从commitLog 中找出未放到消息队列的消息
- SelectMappedBufferResult result = DefaultMessageStore.this.commitLog.getData(reputFromOffset);
- if (result != null) {
- try {
- this.reputFromOffset = result.getStartOffset();
-
- for (int readSize = 0; readSize < result.getSize() && doNext; ) {
- // 对消息做了校验和处理延迟消息,tagCode 放的是延迟时间,方便快速处理,真是牛逼
- DispatchRequest dispatchRequest =
- DefaultMessageStore.this.commitLog.checkMessageAndReturnSize(result.getByteBuffer(), false, false);
- int size = dispatchRequest.getBufferSize() == -1 ? dispatchRequest.getMsgSize() : dispatchRequest.getBufferSize();
-
- if (dispatchRequest.isSuccess()) {
- if (size > 0) {
- // 这里才是重头戏,抓住重点
- DefaultMessageStore.this.doDispatch(dispatchRequest);
-
- if (BrokerRole.SLAVE != DefaultMessageStore.this.getMessageStoreConfig().getBrokerRole()
- && DefaultMessageStore.this.brokerConfig.isLongPollingEnable()
- && DefaultMessageStore.this.messageArrivingListener != null) {
- // long轮询 原来是在这里实时推送消息的
- // 因为客户端只会从主读取消息不会从从读取消息
- DefaultMessageStore.this.messageArrivingListener.arriving(dispatchRequest.getTopic(),
- dispatchRequest.getQueueId(), dispatchRequest.getConsumeQueueOffset() + 1,
- dispatchRequest.getTagsCode(), dispatchRequest.getStoreTimestamp(),
- dispatchRequest.getBitMap(), dispatchRequest.getPropertiesMap());
- notifyMessageArrive4MultiQueue(dispatchRequest);
- }
-
- // TODO-willJo:2021/9/24 这里宕机,上面已经实时推送消息,恢复的时候岂不是就重复推送消息了?客户端会和自己消费的offSet 对比吧?恩,幂等还是很有必要
- this.reputFromOffset += size;
- readSize += size;
- if (DefaultMessageStore.this.getMessageStoreConfig().getBrokerRole() == BrokerRole.SLAVE) {
- DefaultMessageStore.this.storeStatsService
- .getSinglePutMessageTopicTimesTotal(dispatchRequest.getTopic()).add(1);
- DefaultMessageStore.this.storeStatsService
- .getSinglePutMessageTopicSizeTotal(dispatchRequest.getTopic())
- .add(dispatchRequest.getMsgSize());
- }
- } else if (size == 0) {
- this.reputFromOffset = DefaultMessageStore.this.commitLog.rollNextFile(this.reputFromOffset);
- readSize = result.getSize();
- }
- } else if (!dispatchRequest.isSuccess()) {
-
- if (size > 0) {
- log.error("[BUG]read total count not equals msg total size. reputFromOffset={}", reputFromOffset);
- this.reputFromOffset += size;
- } else {
- doNext = false;
- // If user open the dledger pattern or the broker is master node,
- // it will not ignore the exception and fix the reputFromOffset variable
- if (DefaultMessageStore.this.getMessageStoreConfig().isEnableDLegerCommitLog() ||
- DefaultMessageStore.this.brokerConfig.getBrokerId() == MixAll.MASTER_ID) {
- log.error("[BUG]dispatch message to consume queue error, COMMITLOG OFFSET: {}",
- this.reputFromOffset);
- this.reputFromOffset += result.getSize() - readSize;
- }
- }
- }
- }
- } finally {
- result.release();
- }
- } else {
- doNext = false;
- }
- }
- }
- 复制代码
- public void putMessagePositionInfoWrapper(DispatchRequest request, boolean multiQueue) {
- final int maxRetries = 30;
- boolean canWrite = this.defaultMessageStore.getRunningFlags().isCQWriteable();
- for (int i = 0; i < maxRetries && canWrite; i++) {
- long tagsCode = request.getTagsCode();
- if (isExtWriteEnable()) {
- ConsumeQueueExt.CqExtUnit cqExtUnit = new ConsumeQueueExt.CqExtUnit();
- cqExtUnit.setFilterBitMap(request.getBitMap());
- cqExtUnit.setMsgStoreTime(request.getStoreTimestamp());
- cqExtUnit.setTagsCode(request.getTagsCode());
-
- long extAddr = this.consumeQueueExt.put(cqExtUnit);
- if (isExtAddr(extAddr)) {
- tagsCode = extAddr;
- } else {
- log.warn("Save consume queue extend fail, So just save tagsCode! {}, topic:{}, queueId:{}, offset:{}", cqExtUnit,
- topic, queueId, request.getCommitLogOffset());
- }
- }
- boolean result = this.putMessagePositionInfo(request.getCommitLogOffset(),
- request.getMsgSize(), tagsCode, request.getConsumeQueueOffset());
- if (result) {
- if (this.defaultMessageStore.getMessageStoreConfig().getBrokerRole() == BrokerRole.SLAVE ||
- this.defaultMessageStore.getMessageStoreConfig().isEnableDLegerCommitLog()) {
- this.defaultMessageStore.getStoreCheckpoint().setPhysicMsgTimestamp(request.getStoreTimestamp());
- }
- this.defaultMessageStore.getStoreCheckpoint().setLogicsMsgTimestamp(request.getStoreTimestamp());
- if (multiQueue) {
- multiDispatchLmqQueue(request, maxRetries);
- }
- return;
- } else {
- // XXX: warn and notify me
- log.warn("[BUG]put commit log position info to " + topic + ":" + queueId + " " + request.getCommitLogOffset()
- + " failed, retry " + i + " times");
-
- try {
- Thread.sleep(1000);
- } catch (InterruptedException e) {
- log.warn("", e);
- }
- }
- }
-
- // XXX: warn and notify me
- log.error("[BUG]consume queue can not write, {} {}", this.topic, this.queueId);
- this.defaultMessageStore.getRunningFlags().makeLogicsQueueError();
- }
- 复制代码
- read(file, tmp_buf, len);
- write(socket, tmp_buf, len);
- 复制代码
从上图可以看到,总共发生4次上下文切换和4次数据copy
- tmp_buf = mmap(file, len);
- write(socket, tmp_buf, len);
- 复制代码
从上图可以看到,总共发生4次上下文切换和3次数据copy
- sendfile(socket, file, len);
- 复制代码
从上图可以看到,总共发生2次上下文切换和2次数据copy
commitlog 目录下面挂载的文件,在RocketMq 对应的类是MappedFile,我们先看看这个类是怎么创建和初始化的。 当在写入消息的时候,需要判断该文件是否存在
- if (null == mappedFile || mappedFile.isFull()) {
- // 文件写满后,会写新的文件,创建新的文件的时候必定会有毛刺,其实rocketmq 是会提前分配文件来解决这个问题的,并且会在新的文件每一页新0
- // 和上锁(mlock)来确保新文件在虚拟内存中,不至于导致中断回盘带来的性能影响,确实有点牛逼
- mappedFile = this.mappedFileQueue.getLastMappedFile(0); // Mark: NewFile may be cause noise
- }
- 复制代码
mappedFile 初始化
- private void init(final String fileName, final int fileSize) throws IOException {
- this.fileName = fileName;
- this.fileSize = fileSize;
- this.file = new File(fileName);
- this.fileFromOffset = Long.parseLong(this.file.getName());
- boolean ok = false;
-
- ensureDirOK(this.file.getParent());
-
- try {
- /**
- * 这里通过mmap 的技术,来减少用户态和内核态的数据拷贝,从而提成性能(kafka 其实用的是sendfile 技术)
- * 内存映射文件的实际文件写入时机可能是操作系统定期调用,脏页过大,程序主动调用byteBuffer.force,所以后面一个flush 失败的时候不理其实操作系统也会刷进去
- */
- this.fileChannel = new RandomAccessFile(this.file, "rw").getChannel();
- this.mappedByteBuffer = this.fileChannel.map(MapMode.READ_WRITE, 0, fileSize);
- TOTAL_MAPPED_VIRTUAL_MEMORY.addAndGet(fileSize);
- TOTAL_MAPPED_FILES.incrementAndGet();
- ok = true;
- } catch (FileNotFoundException e) {
- log.error("Failed to create file " + this.fileName, e);
- throw e;
- } catch (IOException e) {
- log.error("Failed to map file " + this.fileName, e);
- throw e;
- } finally {
- if (!ok && this.fileChannel != null) {
- this.fileChannel.close();
- }
- }
- }
- 复制代码
创建一个nmap 的代价是很高的,并且刚创建的文件并还没有进行加载到内存里面,依然是在磁盘。这种刚好一个消息进来的时候,文件已经写满了,再去创建一个新的文件,就会出现毛刺,所以RocketMq 采用了提前预热的方式,通过开一个后台线程去扫描mappedFile,发现到达一个配置阈值的时候就去预热下下一个文件
- // pre write mappedFile 这里提前预热文件
- if (mappedFile.getFileSize() >= this.messageStore.getMessageStoreConfig()
- .getMappedFileSizeCommitLog()
- &&
- this.messageStore.getMessageStoreConfig().isWarmMapedFileEnable()) {
- mappedFile.warmMappedFile(this.messageStore.getMessageStoreConfig().getFlushDiskType(),
- this.messageStore.getMessageStoreConfig().getFlushLeastPagesWhenWarmMapedFile());
- }
-
-
- public void warmMappedFile(FlushDiskType type, int pages) {
- long beginTime = System.currentTimeMillis();
- ByteBuffer byteBuffer = this.mappedByteBuffer.slice();
- int flush = 0;
- long time = System.currentTimeMillis();
- for (int i = 0, j = 0; i < this.fileSize; i += MappedFile.OS_PAGE_SIZE, j++) {
- // 每一个页都是写个0
- byteBuffer.put(i, (byte) 0);
- // force flush when flush disk type is sync
- if (type == FlushDiskType.SYNC_FLUSH) {
- if ((i / OS_PAGE_SIZE) - (flush / OS_PAGE_SIZE) >= pages) {
- flush = i;
- mappedByteBuffer.force();
- }
- }
-
- // prevent gc
- if (j % 1000 == 0) {
- log.info("j={}, costTime={}", j, System.currentTimeMillis() - time);
- time = System.currentTimeMillis();
- try {
- Thread.sleep(0);
- } catch (InterruptedException e) {
- log.error("Interrupted", e);
- }
- }
- }
-
- // force flush when prepare load finished
- if (type == FlushDiskType.SYNC_FLUSH) {
- log.info("mapped file warm-up done, force to disk, mappedFile={}, costTime={}",
- this.getFileName(), System.currentTimeMillis() - beginTime);
- mappedByteBuffer.force();
- }
- log.info("mapped file warm-up done. mappedFile={}, costTime={}", this.getFileName(),
- System.currentTimeMillis() - beginTime);
-
- //使用mlock 尽量锁住虚拟内存在物理内存中
- this.mlock();
- }
-
- 复制代码
我们可以看到预热的方式就是往文件里面写0,并且使用了mlock 把内存锁住在物理内存,还是很牛逼的。
这里要明确一点是,sendfile 是不经过用户空间,直接把数据从文件系统通过socket 发送出去的了,但是RocketMq的消费有时候需要根据消息的内容做messageFilter的过滤确认后才能返回给用户,也就意味着这个数据需要经过用户态处理,因此是没有办法使用sendfile 。但是也不是说不能优化,如果没有messageFilter 过滤的是否就意味着可以直接使用sendfile 了呢
- org.apache.rocketmq.store.DefaultMessageStore#getMessage
-
- SelectMappedBufferResult selectResult = this.commitLog.getMessage(offsetPy, sizePy);
- if (null == selectResult) {
- if (getResult.getBufferTotalSize() == 0) {
- status = GetMessageStatus.MESSAGE_WAS_REMOVING;
- }
-
- nextPhyFileStartOffset = this.commitLog.rollNextFile(offsetPy);
- continue;
- }
-
- // 这里把消息取出来后还要进行一层过滤
- if (messageFilter != null
- && !messageFilter.isMatchedByCommitLog(selectResult.getByteBuffer().slice(), null)) {
- if (getResult.getBufferTotalSize() == 0) {
- status = GetMessageStatus.NO_MATCHED_MESSAGE;
- }
- // release...
- selectResult.release();
- continue;
- }